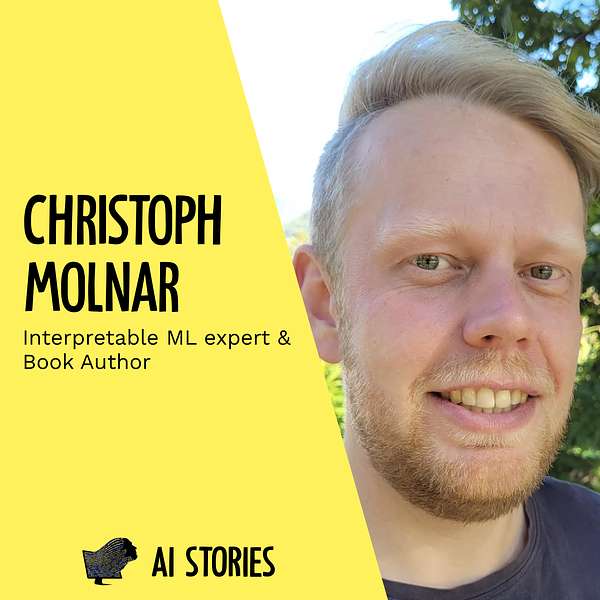
AI Stories
AI Stories
Interpreting Black Box Models with Christoph Molnar #40
Our guest today is Christoph Molnar, expert in Interpretable Machine Learning and book author.
In our conversation, we dive into the field of Interpretable ML. Christoph explains the difference between post hoc and model agnostic approaches as well as global and local model agnostic methods. We dig into several interpretable ML techniques including permutation feature importance, SHAP and Lime. We also talk about the importance of interpretability and how it can help you build better models and impact businesses.
If you enjoyed the episode, please leave a 5 star review and subscribe to the AI Stories Youtube channel.
Link to Train in Data courses (use the code AISTORIES to get a 10% discount): https://www.trainindata.com/courses?affcode=1218302_5n7kraba
Follow Christoph on LinkedIn: https://www.linkedin.com/in/christoph-molnar/
Check out the books he wrote here: https://christophmolnar.com/books/
Follow Neil on LinkedIn: https://www.linkedin.com/in/leiserneil/
---
(00:00) - Introduction
(02:42) - Christoph's Journey into Data Science and AI
(07:23) - What is Interpretable ML?
(18:57) - Global Model Agnostic Approaches
(24:20) - Practical Applications of Feature Importance
(28:37) - Local Model Agnostic Approaches
(31:17) - SHAP and LIME
(40:20) - Advice for Implementing Interpretable Techniques
(43:47) - Modelling Mindsets
(48:04) - Stats vs ML Mindsets
(51:17) - Future Plans & Career Advice